Carmine Rimi
on 9 September 2019
- How To Develop Successful Machine Learning Projects On A Budget – A quick journey through some of the principles for a successful AI getting started project. The article includes an example of how to go from nothing to something – from data pipeline creation to models in production. The primary focus is on a model for hiring and tools you could use, like Kubeflow. How much money will it take to get something off the ground? Read on ..
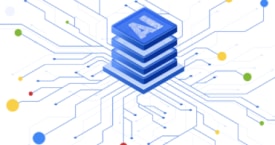
- New homepage and improved collaboration features for AI Hub – Google’s AI Hub is powered by Kubeflow. This announcement discusses new features added to AI Hub. Although still in beta, AI Hub provides a view into how Kubeflow can be leveraged on prem. Do you have platform engineers building your corporate AI toolkit? Read this article to see what Google is doing. Advanced multi-user use cases, new machine learning taxonomy, asset favoriting, and public data sets or solutions – including a TensorRT-optimized BERT notebook from Nvidia.
- Three pitfalls to avoid in machine learning – As scientists from myriad fields rush to perform algorithmic analyses, Google’s Patrick Riley calls for clear standards in research and reporting. Machine-learning tools can turn up fool’s gold — false positives, blind alleys and mistakes. Read this article to learn about three problems in machine-learning analyses that Google faced, and solved, in the Google Accelerated Science team.
- Use case spotlight: Little Ripper deploys croc-spotting AI drones – A different take on how AI is saving lives. To help keep beachgoers safe from crocodiles 🐊 in the water and on land, the same AI drone technology that the Little Ripper Group used for its shark 🦈 detection drones is now being used to spot crocodiles in Queensland. In additino to spotting wildlife, this technology is used to help swimmers. Last summer, 51 drones were deployed around Australia to help spot rips and swimmers in distress.
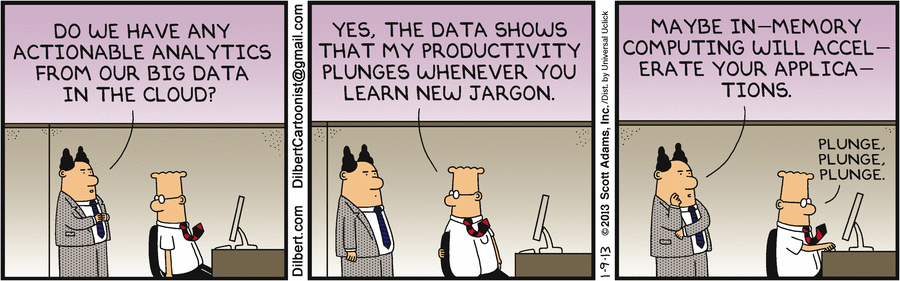